Limitations of the least squares estimators; a teaching perspective
Citation
O’Driscoll, D. and Ramirez, D.E. (2016). "Limitations of the Least Squares Estimators; A Teaching Perspective", Athens: ATINER'S Conference Paper Series, No: STA2016-2074.
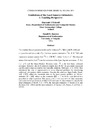
View/ Open
Date
2016Author
O'Driscoll, Diarmuid
Ramirez, Donald E.
Peer Reviewed
NoMetadata
Show full item record
O’Driscoll, D. and Ramirez, D.E. (2016). "Limitations of the Least Squares Estimators; A Teaching Perspective", Athens: ATINER'S Conference Paper Series, No: STA2016-2074.
Abstract
The standard linear regression model can be written as Y = Xβ+ε with X a full rank n × p matrix and L(ε) = N(0, σ2In). The least squares estimator is = (X΄X)−1XY with variance-covariance matrix Coυ( ) = σ2(X΄X)−1, where Var(εi) = σ2. The diagonal
terms of the matrix Coυ( ) are the variances of the Least Squares estimators 0 ≤ i ≤ p−1 and the Gauss-Markov Theorem states is the best linear unbiased estimator. However, the OLS solutions require that (X΄X)−1 be accurately computed and ill conditioning can lead to very unstable solutions. Tikhonov, A.N. (1943) first introduced the idea of regularisation to solve ill-posed problems by introducing additional information which constrains (bounds) the solutions. Specifically, Hoerl, A.E. (1959) added the constraint term to the least squares problem as follows: minimize ||Y – Xβ||2 subject to the constraint ||β||2 = r2 for fixed r and dubbed this
procedure as ridge regression. This paper gives a brief overview of ridge regression and examines the performance of three different types of ridge estimators; namely the ridge estimators of Hoerl, A.E. (1959), the surrogate estimators of Jensen, D.R. and Ramirez, D.E. (2008) and the raise estimators of Garcia, C.B., Garcia, J. and Soto, J. (2011).
Keywords
LimitationsLeast
Squares
Estimators
Teaching perspective