Mitigating collinearity in linear regression models using ridge, surrogate and raised estimators
Citation
O'Driscoll, D., Ramirez, D.E. (2016) 'Mitigating collinearity in linear regression models using ridge, surrogate and raised estimators.' Cogent Mathematics 3(1144697), pp.1-9. DOI: 10.1080/23311835.2016.1144697.
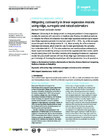
View/ Open
Date
2016Author
O'Driscoll, Diarmuid
Ramirez, Donald E.
Peer Reviewed
YesMetadata
Show full item record
O'Driscoll, D., Ramirez, D.E. (2016) 'Mitigating collinearity in linear regression models using ridge, surrogate and raised estimators.' Cogent Mathematics 3(1144697), pp.1-9. DOI: 10.1080/23311835.2016.1144697.
Abstract
Collinearity in the design matrix is a frequent problem in linear regression models, for example, with economic or medical data. Previous standard procedures to mitigate the effects of collinearity included ridge regression and surrogate regression.
Ridge regression perturbs the moment matrix X'X → X'X + kIp, while surrogate regression perturbs the design matrix X → Xs. More recently, the raise estimators have been introduced, which allow the user to track geometrically the perturbation
in the data with X→XX. The raise estimators are used to reduce collinearity in linear regression models by raising a column in the experimental data matrix, which may be nearly linear with the other columns, while keeping the basic OLS regression
model. We give a brief overview of these three ridge-type estimators and discuss practical ways of choosing the required perturbation parameters for each procedure.
Keywords
CollinearityRidge estimators
Surrogate estimators
Raise estimators